AI is everywhere, changing how we live, work, and play. But not all AI is created equal. Ever wondered about the difference between generative AI vs predictive AI? Let’s break it down and see what sets them apart.
Artificial Intelligence, or AI, is the magic behind everything from smart assistants to self-driving cars. It’s a tech marvel that’s reshaping industries and everyday life.
According to a recent study, the AI market is expected to grow from $196.63 billion in 2023 to approximately $950 billion by 2028. That’s a lot of growth driven by different types of AI doing different things.
Therefore, understanding the various types of AI is crucial. Because knowing the difference helps us harness the power of AI more effectively. It’s like knowing the difference between a sports car and a pickup truck, you wouldn’t use one for the job of the other. The same goes for AI.
So, let’s dive into the fascinating world of AI by comparing generative AI vs predictive AI. What are they? How do they work? And why should you care? We’ve got all the answers lined up for you.
Understanding Generative AI
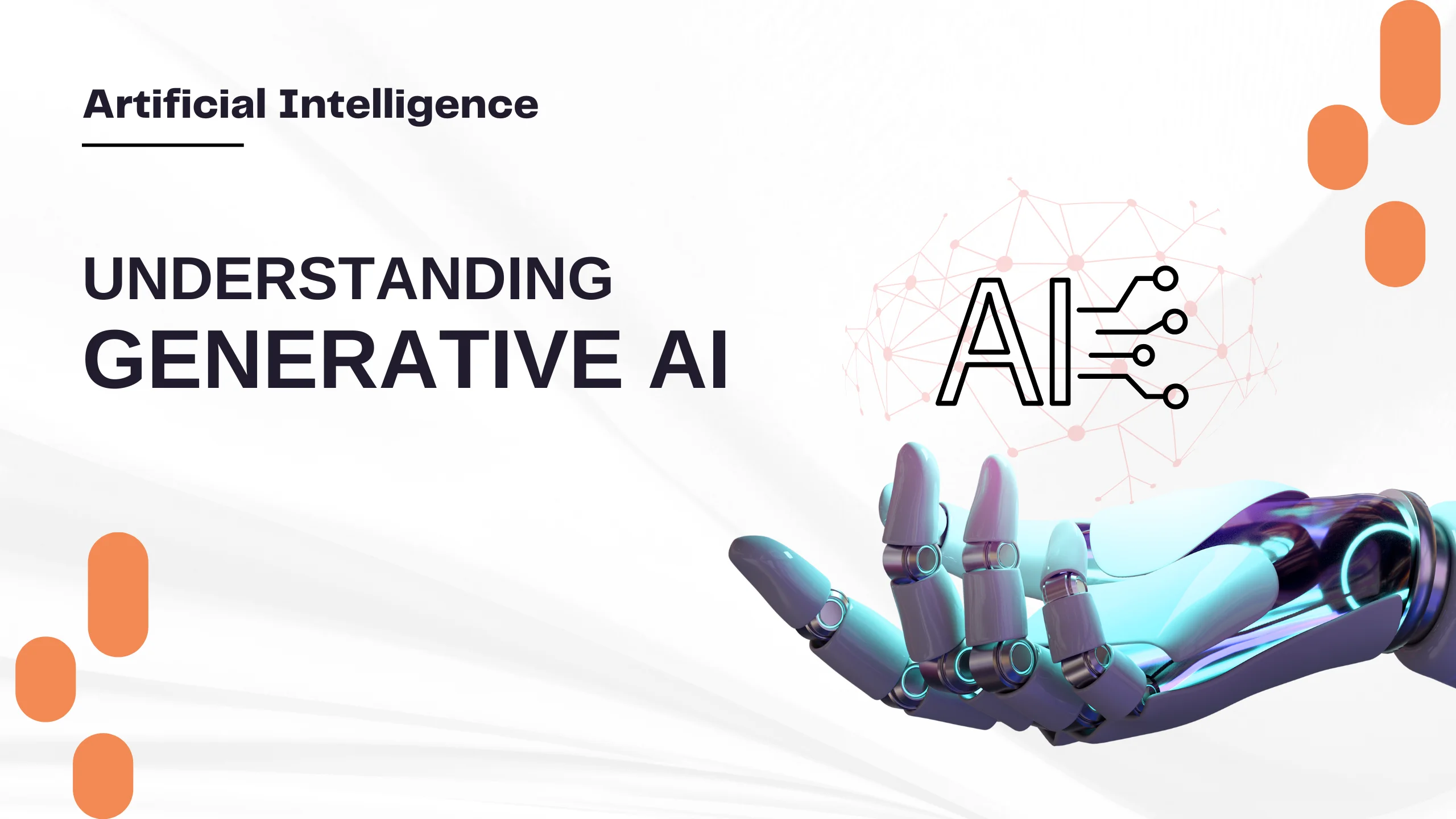
Unveiling the Magic of Generative AI: A Deep Dive into Creative Technology
Generative AI refers to a type of artificial intelligence that can create new content based on the data it has been trained on. It uses models that learn from a vast amount of information. Moreover, it then generates new data that mirrors the patterns and styles of the original data.
Furthermore, the core principles revolve around neural networks, specifically a type known as generative adversarial networks (GANs) and transformers. Additionally, these models work by pitting two neural networks against each other (in the case of GANs) or by predicting the next word in a sentence (in the case of transformers).
Examples of Generative AI Models
- GPT (Generative Pre-trained Transformer): Developed by OpenAI, GPT is one of the most well-known generative AI models. It can write essays, answer questions, summarize texts, and even create poetry. Furthermore, GPT-3, has 175 billion parameters, making it incredibly powerful and versatile.
- DALL-E: Also from OpenAI, DALL-E is a model that generates images from textual descriptions. For example, tell it to draw “a two-story pink house shaped like a shoe,” and it will produce a unique image that matches your description.
Benefits of Generative AI
Generative AI technology offers significant benefits across a wide range of industries:
- Enhanced Creativity: Generative AI allows for the creation of new and innovative content, spanning from art to literature, transforming creative processes.
- Personalization: It enables customized recommendations and experiences for users, enhancing customer satisfaction and engagement.
- Efficiency: Automating repetitive tasks frees up human resources for more strategic endeavors.
- Cost Savings: Reduces operational costs through automation, leading to significant financial benefits.
- Data Synthesis: Generates insights from vast datasets, extracting patterns and trends that humans might overlook.
Challenges and Limitations of Generative AI
Despite its potential, generative AI faces several challenges and limitations.
- Ethical Concerns: Raises ethical dilemmas regarding the use of AI-generated content and potential biases.
- Quality Control: Ensuring the quality and accuracy of AI-generated outputs remains a persistent challenge.
- Security Risks: Vulnerabilities in AI systems can be exploited, posing security threats such as data breaches and malicious use.
- Dependency on Data: This relies heavily on the quantity and quality of training data, which can limit its applicability.
- Regulatory Hurdles: Compliance with evolving regulatory frameworks can be complex and restrictive.
What is Predictive AI?
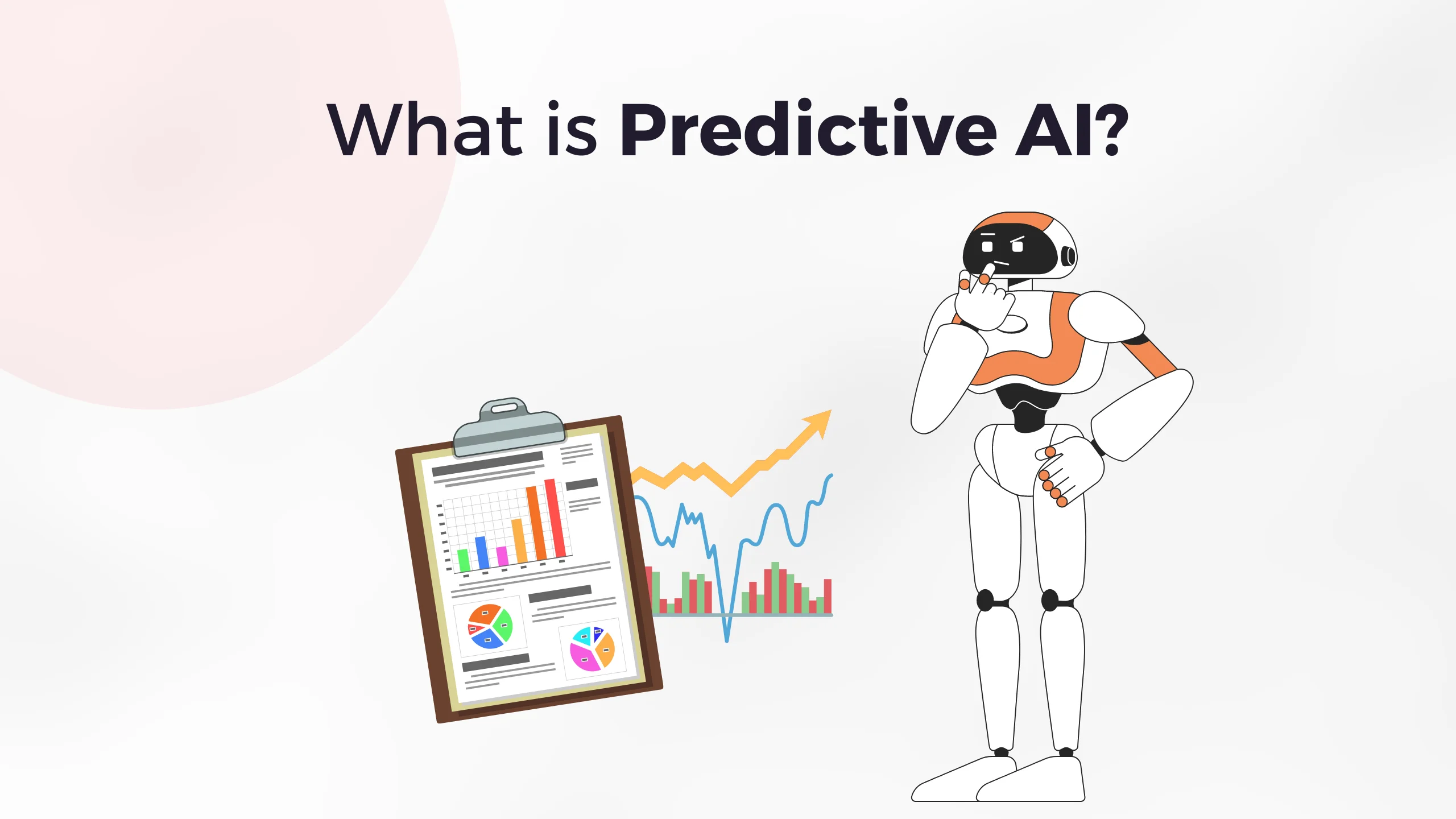
Decoding Predictive AI: Harnessing Data to Predict the Future
Predictive AI refers to artificial intelligence that uses historical data to make predictions about future events. It relies on various statistical techniques and machine learning models to identify patterns and trends in the data. Moreover, unlike generative AI, which creates new content, predictive AI focuses on using existing data to predict what’s coming next.
Examples of Predictive AI Models
- Regression Models: These are among the simplest and most widely used predictive models. Linear regression, for instance, predicts a dependent variable based on the value of one or more independent variables.
For example, it can forecast sales based on advertising spend.
- Neural Networks: More complex than regression models, neural networks are designed to recognize patterns in large datasets. As a result, they are used in a variety of predictive tasks, from stock market forecasting to predicting consumer behavior.
Benefits of Predictive AI
Predictive AI provides notable benefits in predictive analytics and decision-making processes.
- Forecasting Accuracy: Provides highly accurate predictions based on historical data, improving strategic planning and risk management.
- Enhanced Insights: Uncovering hidden patterns and correlations within data leads to actionable insights and informed decisions.
- Optimized Operations: Streamlining processes by predicting maintenance needs, demand fluctuations, and resource allocation.
- Customer Satisfaction: Anticipates customer preferences and behavior, enabling personalized marketing strategies.
- Competitive Edge: Provides a competitive advantage by enabling proactive rather than reactive decision-making.
Challenges and Limitations of Predictive AI
However, predictive AI faces several challenges that need to be addressed:
- Data Quality: Relies heavily on the quality and relevance of historical data for accurate predictions.
- Overfitting Risks: Models may overfit specific datasets, leading to inaccurate predictions when applied to new data.
- Interpretability: Understanding the reasoning behind AI-generated predictions can be challenging, affecting trust and adoption.
- Bias and Fairness: Like Generative AI, Predictive AI can perpetuate biases present in historical data, leading to unfair outcomes.
- Implementation Complexity: Integrating predictive models into existing systems and workflows can be technically challenging and resource-intensive.
Generative AI vs Predictive AI: Core Differences
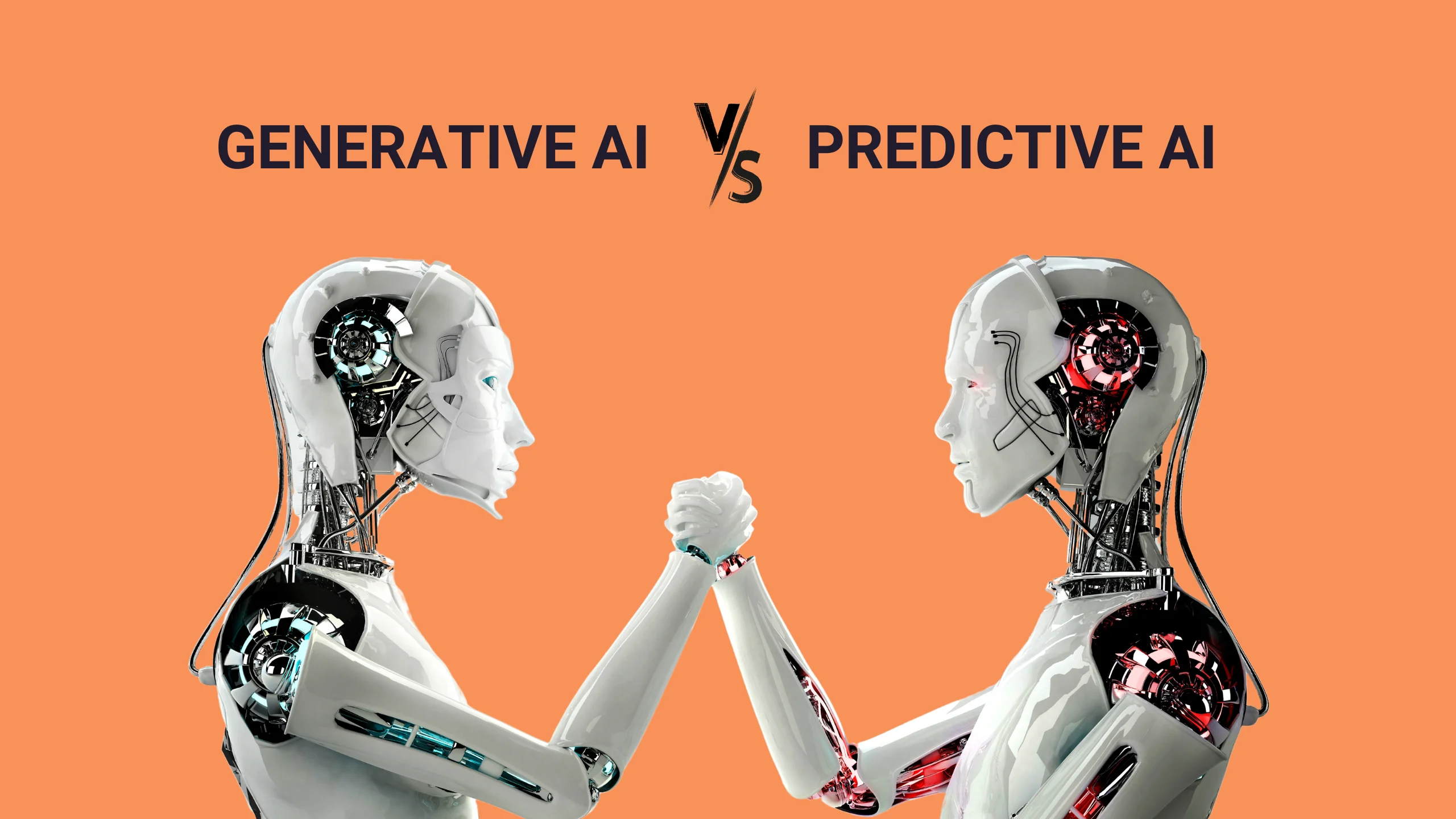
Generative AI vs Predictive AI
Unraveling the Distinctions: Generative AI vs Predictive AI
Aspect | Generative AI | Predictive AI |
Functionalities | Generates text, images, music, and other creative content | Predicts trends, behaviors, and events |
Technical Distinctions | Uses models like GANs and transformers for training on large datasets to create new outputs | Uses models like regression, and neural networks to identify patterns. Moreover, it also uses decision trees. |
Data Usage | Trains on vast amounts of data to understand and replicate patterns | Analyzes historical data to find trends and correlations |
Applications |
|
|
Evaluation Criteria | Subjective evaluation of creativity and realism | Statistical accuracy and reliability of predictions |
Roles | Explores new possibilities in creative fields | Provides recommendations and actions based on predictions |
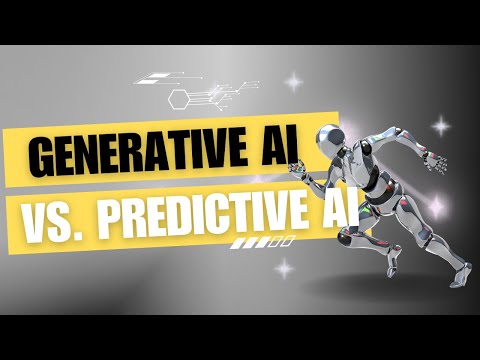
In comparing generative AI vs predictive AI, it’s clear that both types of AI serve distinct purposes and offer unique benefits. Generative AI excels in creative and exploratory applications. Predictive AI, on the other hand, shines in analytical and prescriptive roles.
Generative AI vs Predictive AI: Case Studies
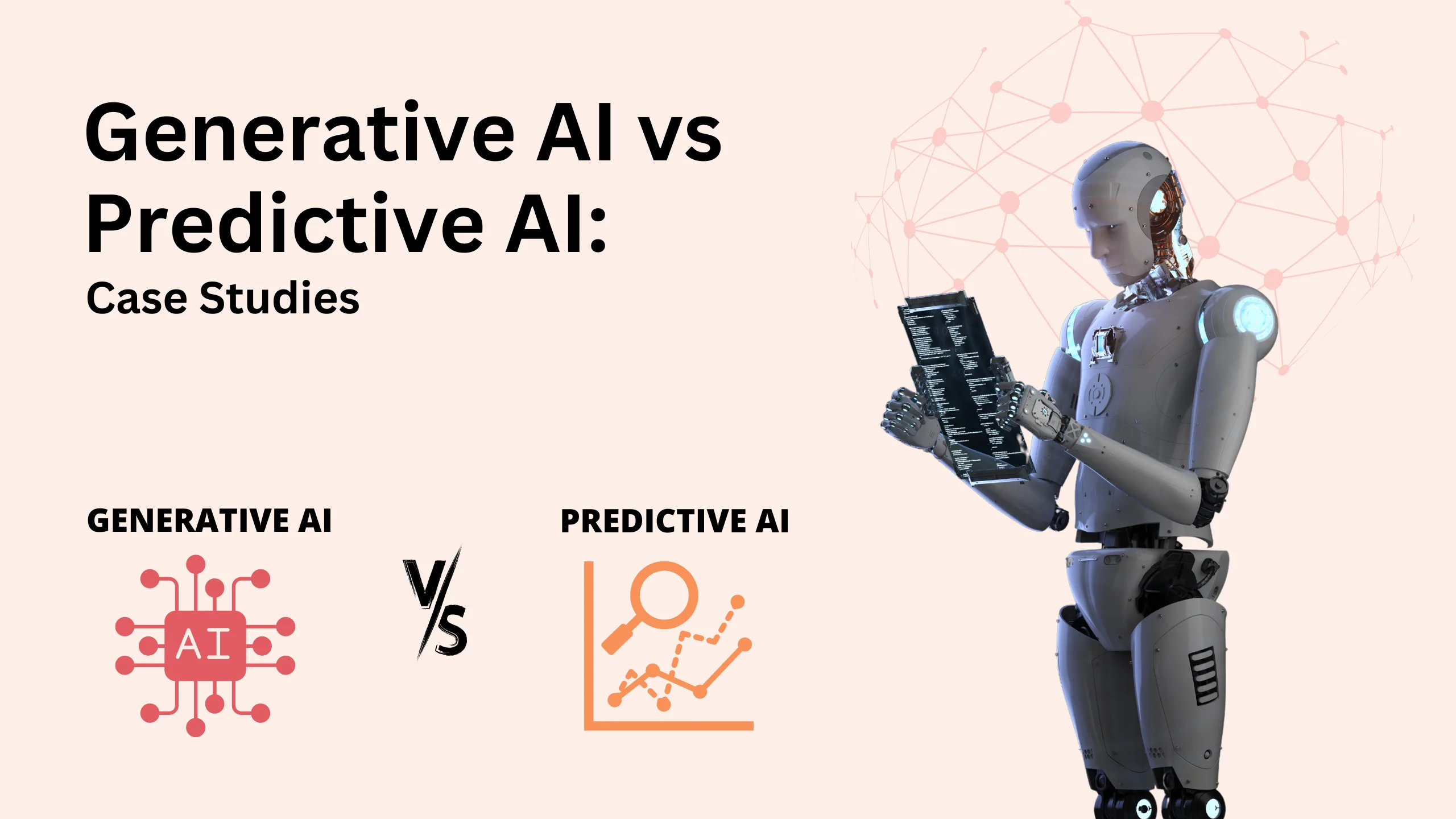
Exploring Real-world Applications: Case Studies of Generative AI vs Predictive AI
To truly grasp the impact and capabilities, examining generative AI vs predictive AI examples is essential. Therefore, let’s explore a couple of notable examples that highlight the strengths and practical uses
OpenAI’s GPT-4
OpenAI’s GPT-4 is one of the most advanced generative AI models to date. It has been trained on a diverse range of internet text to generate human-like text based on the input it receives. As a result, GPT-4 can perform various language tasks, including writing essays, summarizing texts, translating languages, and even generating code.
Outcome:
- Increased Efficiency: Firstly, businesses can produce high-quality content quickly. Thus, this reduces the time spent on writing and editing.
- Enhanced Creativity: GPT-4’s ability to generate diverse content helps marketers explore new ideas and approaches.
- User Satisfaction: Lastly, the platform has received positive feedback for its ease of use and the quality of the generated content.
Netflix’s Recommendation System
Netflix utilizes predictive AI to enhance its recommendation system. Netflix’s system collects data on user viewing habits, such as watch time, ratings, and interactions with content.
Additionally, using this data Netflix’s algorithms predict what movies or TV shows a user might enjoy. As a result, this improves user engagement and satisfaction.
Outcome:
- Improved User Retention: Firstly, personalized recommendations keep users engaged and reduce churn rates.
- Increased Watch Time: Users spend more time on the platform, exploring new content that aligns with their tastes.
- Enhanced User Experience: Lastly, tailored content recommendations provide a more enjoyable and relevant viewing experience.
Generative AI vs Predictive AI: Future Trends
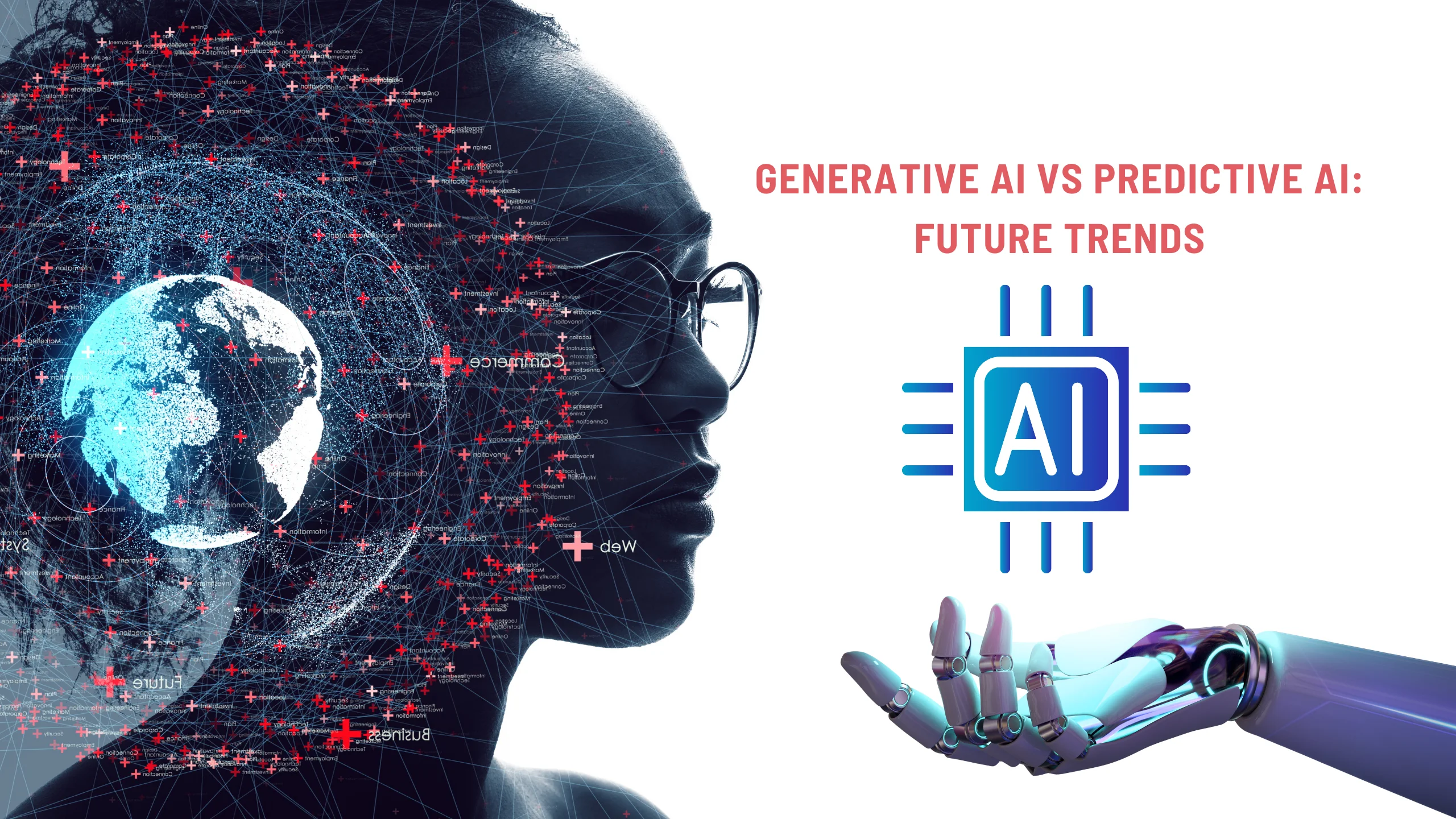
Shaping Tomorrow: Future Trends in Generative AI vs Predictive AI
The future of AI is evolving at an incredible pace, with generative AI vs predictive AI leading the charge in transforming various industries. Therefore, it’s time to explore the emerging trends and innovations that will shape the future of Generative AI vs Predictive AI.
Future Trends in Generative AI:
- Enhanced Personalization: Firstly, from tailored marketing messages to custom artwork, AI-generated content will become more nuanced and aligned with individual preferences.
- Improved Human-AI Collaboration: Moreover, as generative AI models become more sophisticated, the collaboration between humans and AI will deepen.
- Advances in Natural Language Understanding: Additionally, future iterations of models like GPT will facilitate more natural and engaging interactions with AI that are more effective and human-like.
Future Trends in Predictive AI:
- Increased Accuracy: AI for predictive analytics will continue to improve in accuracy and reliability as algorithms become more advanced and datasets grow larger.
- Integration with IoT and Big Data: The integration of predictive AI with the Internet of Things (IoT) and big data analytics will unlock new possibilities. As a result, this convergence will lead to more efficient and responsive systems.
- Real-Time Predictions and Adaptability: The future of AI predictive lies in real-time predictions and adaptability. Therefore, AI systems will become capable of making instant predictions and adjusting their models on the fly based on new data.
How Insnapsys Helps You Compare Generative AI vs Predictive AI
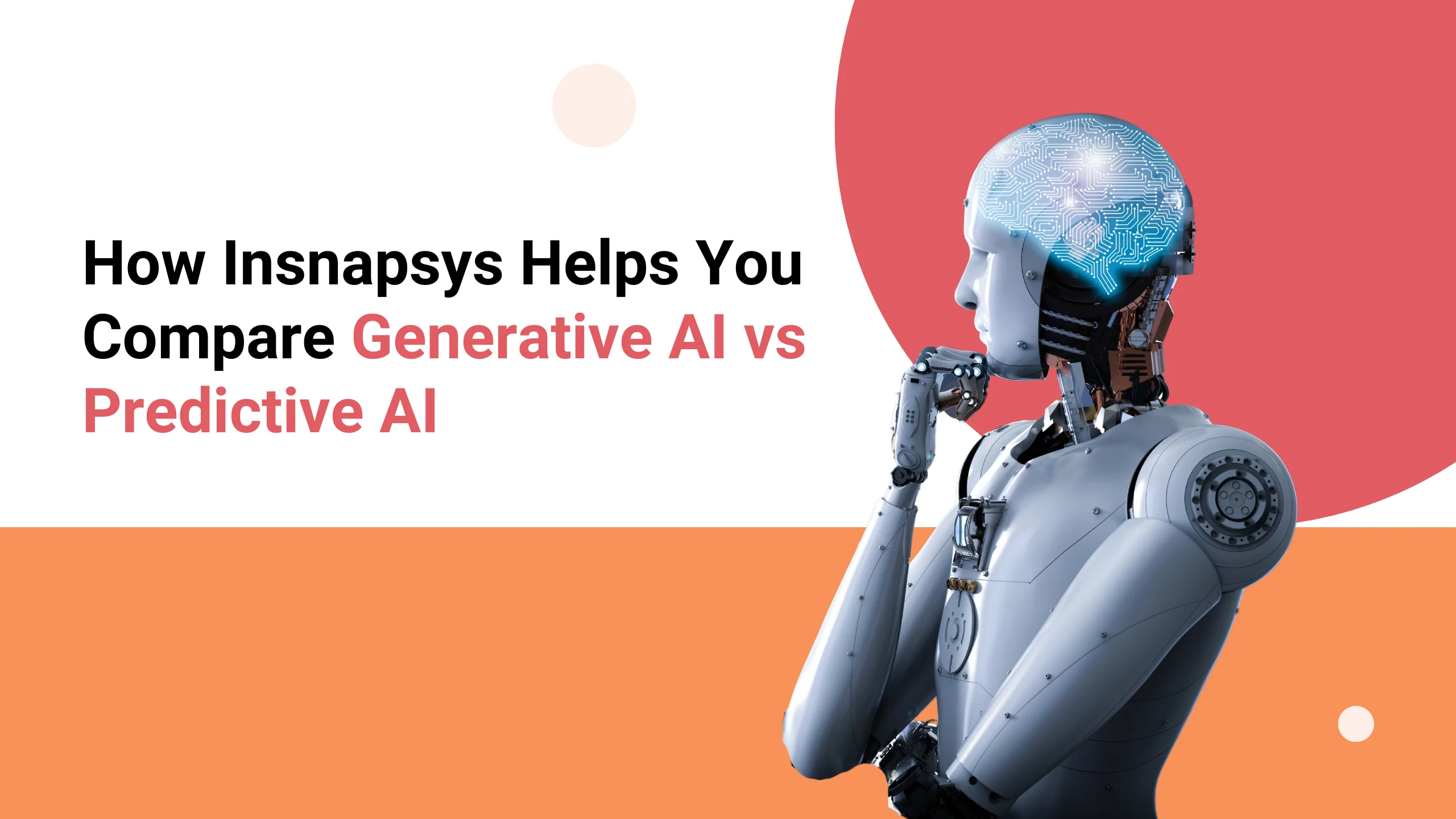
Navigating AI Choices: How Insnapsys Simplifies Generative AI vs Predictive AI Comparisons
Insnapsys is a premier IT staffing company that specializes in providing top-tier developers tailored to meet the unique requirements of businesses. Therefore, let’s look at how Insnapsys helps companies navigate the complexities of generative AI vs predictive AI by providing the right talent.
- Understanding Your Needs: Firstly, Insnapsys begins by understanding the specific needs of your business. Moreover, they assess whether your projects would benefit more from generative AI, predictive AI, or a combination of both.
- Detailed Comparison: Secondly, Insnapsys helps businesses understand the core principles, functionalities, and applications of generative AI vs predictive AI.
- Thorough Screening: Furthermore, Insnapsys conducts rigorous screening processes to ensure that the developers they provide meet the highest standards.
- Generative AI Experts: For businesses focused on creative content generation, Insnapsys sources developers skilled in generative AI technologies.
- Predictive AI Specialists: If your business needs to forecast trends and behaviors, Insnapsys provides developers experienced in predictive AI.
- Continuous Improvement: Lastly, Insnapsys ensures that the developers they provide continue to meet your evolving needs and that you can manage a remote team.
Also Read: The Intersection of Cloud Computing and AI
Conclusion: Generative AI vs Predictive AI
Understanding the comparison of generative AI vs predictive AI is crucial for harnessing the full potential of artificial intelligence. On one side, generative AI excels in creating new and innovative content, while predictive AI focuses on analyzing historical data to forecast future trends and behaviors.
In short, by examining case studies and future trends, it becomes clear that both types of AI offer unique benefits and applications. Moreover, companies like Insnapsys play a pivotal role in helping businesses navigate these technologies by providing the right talent and expertise.
Therefore, as AI continues to evolve, the rivalry of generative AI vs predictive AI will drive further advancements, transforming how we create, analyze, and innovate.
FAQs
-
Which best describes the difference between predictive AI and generative AI?
Generative AI creates new content, like text, images, or music, from scratch. Predictive AI, on the other hand, analyzes past data to make predictions about the future.
-
How is generative AI different from predictive AI?
Generative AI makes new things, like a writer creating a story. On the other hand, Predictive AI looks at old information to guess what might happen next, like a weather forecast.
-
How does predictive AI work?
Predictive AI works by examining lots of past data to find patterns. Additionally, it then uses these patterns to make guesses about what will happen in the future.
-
Can generative AI make predictions?
No, generative AI is not designed to make predictions. However, predictive AI is the type that makes forecasts based on past data.
-
Is Alexa a generative AI?
No, Alexa is not a generative AI. Alexa uses predictive AI and other technologies to understand and respond to your voice commands.
-
What is the difference between AI vs generative AI?
Artificial Intelligence (AI) is a broad field encompassing machines that can perform tasks that typically require human intelligence, such as problem-solving or learning from data. Generative AI, on the other hand, refers to a subset of AI focused specifically on creating new content, often in the form of text, images, or even music, that mimics human creativity.